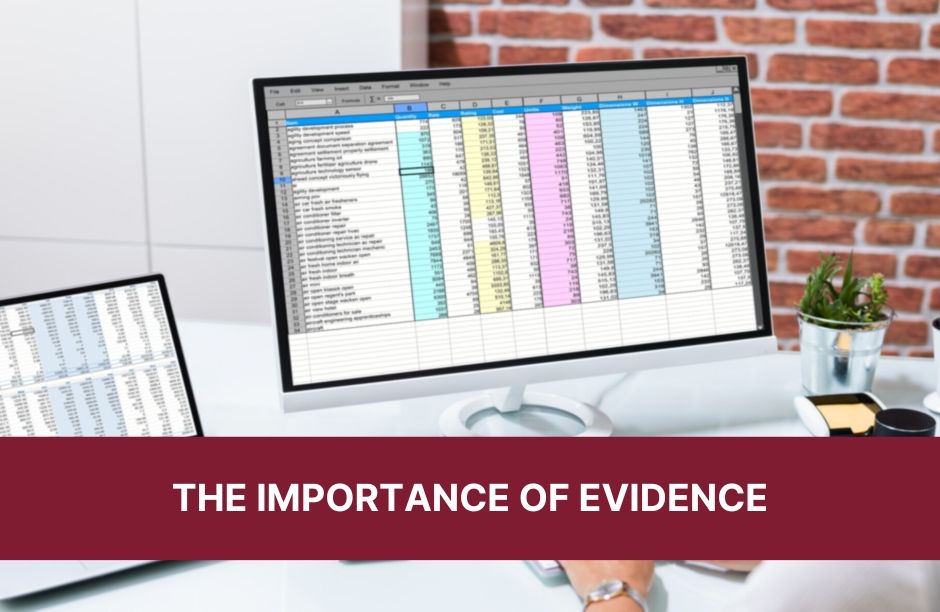
“Insofar as a scientific statement speaks about reality, it must be falsifiable; and in so far as it is not falsifiable, it does not speak about reality.” - Karl Popper Any money spent on an intervention that doesn’t work is money wasted. Money that could have saved lives. This is why evidence is so important - you’ve got to have a high level of confidence that the intervention is having the intended effect.
Imagine two charities: Stop AIDS (a made-up charity for illustration’s sake), and Homeopaths Without Borders (a real charity, unfortunately). Stop AIDS provides antiretroviral drugs, which are clinically-proven to dramatically improve the quality and quantity of life of HIV positive patients. Homeopaths Without Borders provides ‘medicine’ that has been proven ineffective. Now, we chose an obviously ineffective charity to prove our point, but there are many other charities that have no scientific evidence of their impact. In fact, it’s the norm. And there are a fair few charities out there that have been evaluated and deemed harmful, yet they’re still actively seeking and accepting funding. So, how can you identify good evidence of effectiveness? Your best bet is to look for controlled studies, with an emphasis on falsifiability and a convergence of evidence.
LOOK FOR CONVERGENCE
Convergence is a very important but oft-forgotten concept. In short, the more diverse pieces of evidence that converge on the same conclusion, the more confident we can be about that conclusion. This is because if they are indeed independent sources of evidence, it’s unlikely that they would converge on the same conclusion unless it were true. Convergence suggests robustness - the idea that a claim holds true even when you look at it from a different angle. Such ambiguities ought to concern any charity founder. How can we be sure some intervention will work in one place, just because it worked somewhere else? Many people place too much weight on one strong study, changing their minds too rapidly, even if previous weaker studies or macro-level data point the other way.
FALSIFIABILITY
What is falsifiability?
A claim is ‘falsifiable’ if there is evidence that could prove it does not work. In other words, we can make a prediction and test it, and if the prediction is inaccurate, then the claim is proven to be false. However, if a claim is ‘unfalsifiable’, that means it can never be proven or disproven.
How can you spot an ‘unfalsifiable’ intervention or charity?
‘Unfalsifiable’ charities ignore any evidence against their effectiveness or explain it away with post hoc rationalizations.
For example, many studies have shown that microloans don’t increase income, and it’s better to give the poor money with no strings attached. However, microloan charities still exist and either ignore this evidence, or say that the real point of microloans is to empower women. When more evidence comes out casting doubt on whether they are even successful at that, they say the point of their services is actually to smooth out income fluctuations. This claim might be true, but at this stage you should smell a rat - you’d need to wait to find evidence supporting this, and it’s starting to look like microloans are not all they’re cracked up to be.
Note that microloans are technically falsifiable, but psychologically not so. They did indeed make claims that were disproven. Nonetheless, the proponents either refused to see the evidence, or moved the goalposts, making it essentially unfalsifiable.
What’s the problem with unfalsifiable charities?
Just as scientists had to accept that the world is round, charities must learn to admit when they realize their interventions aren’t working. We can never improve the world if we refuse to acknowledge and learn from our mistakes.
How can you make sure your intervention is falsifiable? There are four main ways to hold yourself accountable to the evidence:
Make sure you can clearly define success and failure, so you cannot weasel out of failures later by saying you were trying to accomplish something different anyway.
Counter confirmation bias by actively trying to prove your intervention wrong.
Be prepared to abandon or change your intervention if it doesn’t work. It helps to have a Plan B you’re excited about, so that you do not feel like the world will end if Plan A doesn’t work out.
Get an external review. It's very hard to evaluate the evidence objectively, but an intelligent third party can be much more impartial than you. (GiveWell is a good example of this.)
AN EXEMPLARY FALSIFIABLE CHARITY: GIVEDIRECTLY
Take GiveDirectly as an example of a charity that's as falsifiable as they come. They give money to the poorest people in Kenya and Uganda, no strings attached. There are 11 randomized controlled trials proving that this intervention improves long term income, empowers women, and makes people happier, which we believe is the ultimate metric for a charity’s success.
Not only that, but one of the studies was run by GiveDirectly themselves, and they publicly defined what they would regard as success or failure, explained how they would analyze the data, and precommitted to publishing the results regardless. This was a bold and admirable move. They could easily have found that the data did not confirm their intervention’s success.
You can see their dedication to transparency even in their marketing. In one of their social media campaigns, they told stories about what people spent the money on. Unlike the vast majority of other charities though, they did not cherry-pick the most compelling stories. They randomly selected them, so the examples were truly representative.
GiveDirectly is one of the very few falsifiable charities that goes to great lengths to test itself. That means you can trust them to actually do what they say they will.
COMMONLY USED TYPES OF EVIDENCE
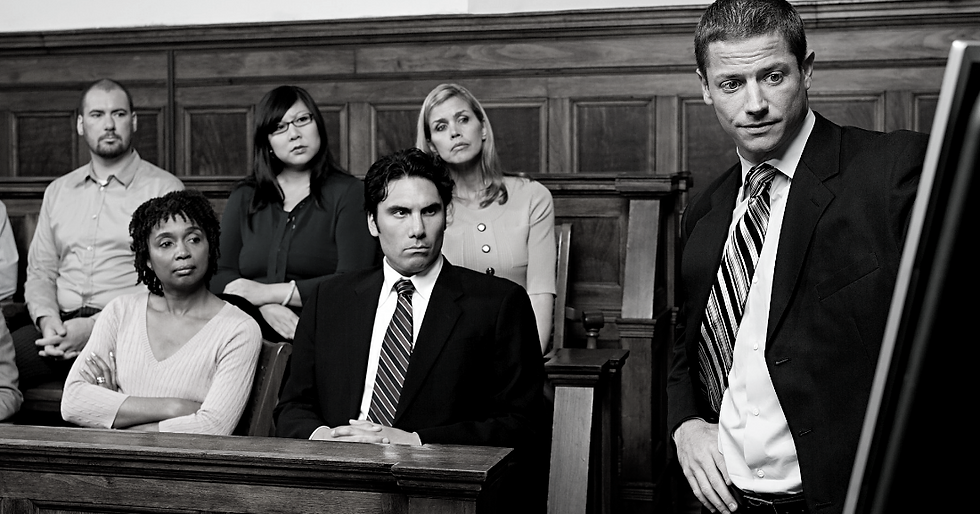
There are thousands of different types of evidence ranging from casual anecdotes to randomized controlled trials with hundreds of thousands of participants. How can you know which sources to trust when researching interventions? We summarise some of the key pros and cons of the types of evidence charities use most commonly, in order from strongest to weakest.
Meta-analyses and systematic reviews
Meta-analyses and systematic overviews are at the top of the evidence pyramid. These involve systematically identifying all the studies that satisfy predefined criteria, such as strength of study or target population. They then analyse the dataset and determine which conclusion is best supported by all the currently available experiments. General standards for meta-analyses and systematic reviews make them less prone to bias than simply perusing the existing literature according to interest and coming to a conclusion at an arbitrary point.
This is a huge part of the reason we, the authors, like the work done by organizations like GiveWell, as they can look at large numbers of studies and put together important overviews that allow others to easily and painlessly come to an informed opinion.
Individual scientific studies
Generally, scientific studies are very reliable sources of information. In the medical sciences, studies are necessary to determine whether a new drug is working. For charities, studies are equally important to prove whether the charity is having a real positive impact. However, studies can vary widely in strength from randomized control trials (RCTs) to much weaker observational studies. Studies vary in quality, so do not take the results at face value, but rather evaluate each study based on its own merits.
Expert opinions
The reliability of ‘expert opinions’ is entirely dependent on who the expert is and the field they are in. For example, a recommendation from a prominent expert in a relevant field is usually much more trustworthy than a recommendation from an academic who specializes in another subject.
You must also try to gauge whether the expert is biased. For example, academics tend to favor their own fields and their own work within them. A researcher who specializes in vitamin A supplements may think that their cause area is the most important and another researcher in education will think the same, though both do not have much understanding of the other’s field. While they may know within their area which is the best intervention, they will be hard pressed to compare between areas.
This natural bias can be used to your advantage, however. When considering whether to implement an intervention, try running your idea past several reasonable outsiders whose values and epistemologies you respect. If many of them give you negative feedback, you can be pretty sure that the idea isn’t as good as you thought, because the vast majority of people tend towards over-optimism, or at least try to avoid hurting your feelings by being positive.
Additionally, some subjects give better expert advice than others according to how tractable the subject is. A mechanic, for instance, will likely know exactly what to do to fix a car. Cars have limited moving parts and each part is understood well, with clear and quick feedback loops. On the other hand, if you ask ten futurists what will happen in 50 years you will get ten different answers. There are countless moving parts, each of which is poorly understood, and feedback loops are muddy and slow. While most of the charitable areas you will likely consider do not have the advantage of having such a well understood field as car mechanics, some fields still do have more reliable experts than others. For example, health experts are more reliable than experts on social change, which is a much messier and less well understood field.
Be aware that, while your source may have some valuable insights, it’s also equally possible that his or her recommendation could be worthless. Even if you think your recommender is genuinely reputable, you should be sure to question their original sources. Is their opinion based on personal stories and reasoning, or more solid evidence like studies and systematic overviews? If at all possible, rely on the primary sources that the expert is using rather than the opinion of the expert themselves. Expert opinion should generally be used as a jumping off point or as a resource if you are not planning on putting much time into the area, rather than a primary source for choosing a charity to start.
Historical evidence
Some people use historical evidence to predict which interventions will work and which might require more thorough testing. Sadly, there are significant problems with most historical evidence. In practice, most charities’ historical evidence is largely unreliable and cherry-picked to support a predetermined conclusion.
Some general characteristics of good and bad historical evidence are listed below:

A priori reasoning A priori reasoning is based on logic or common sense. For example, “Women in developing countries have to collect water at pumps, which is long and difficult. Children have a lot of pent up energy but no playgrounds. If we make a merry-go-round that pumps water, the children will have a place to play and run around while simultaneously getting water and the women will be free to follow other pursuits.” Logical or common sense reasoning can feel more reliable than personal stories, but it suffers from many of the same problems. The reliability of a priori reasoning depends partly on the field of research. For example, reasoning in mathematics is more useful than historical evidence, but historical evidence in sociology is more useful than a priori reasoning. In the charity sector, the world is messy and rarely goes according to plan. For that reason, you should not rely on this kind of evidence alone to evaluate charities and interventions. When lives are at stake, armchair reasoning is not enough. Anecdotes and personal stories Anecdotes and personal stories are the weakest forms of evidence and, sadly, the most commonly used in the charity sector. They are almost always cherry-picked and unrepresentative of the norm. You should never rely on them as your sole form of evidence for an intervention’s effectiveness. However, that’s not to say that personal stories don’t have a place in the charity sector. Good personal stories can be extremely compelling emotionally, and they can be a great way to attract donations for a cause that other evidence already supports.
GETTING THE EVIDENCE YOURSELF It’s often a good idea to run an RCT on your own intervention, even if there’s already strong evidence that it has been effective in the past. You might be running a slightly different intervention or working in a different context than previous experiments. You may also want to measure metrics that other RCTs do not typically measure, such as subjective well-being, or look into any flow-through effects that may concern you.
THE CASE FOR TESTING NEW IDEAS WITHOUT ANY EVIDENCE - YET
Under the right circumstances, testing new and innovative ideas can be very valuable. For example, testing allows you to share your results with other charities, which can then learn from your mistakes or replicate your successes. Without testing new unproven concepts, we may never find the most effective interventions. And in this circumstance, settling for less than the ‘best’ intervention means accepting the fact your target population will suffer more, or for a longer period of time. Of course, not every idea is worth trying and we wouldn’t usually recommend taking a chance on an intervention with zero evidence to suggest it may work. We believe the most promising interventions for trial and error have some prior evidence suggesting they may be important, but not enough evidence to answer all important questions. If the intervention has a foundation of suggestive positive evidence then the chance that your research will turn out to be totally useless or irrelevant is lower. Time You should weigh the existing evidence for the innovation against the resources required to test it. For example, if an idea only takes a day to test, we require much weaker evidence to try it than if the test takes six months of work. Expected value If the intervention has extremely high expected value, then the risk will be more favourable. We can compromise on evidence because the potential payoff would be astronomical.
TRICKS PEOPLE PLAY WITH EVIDENCE
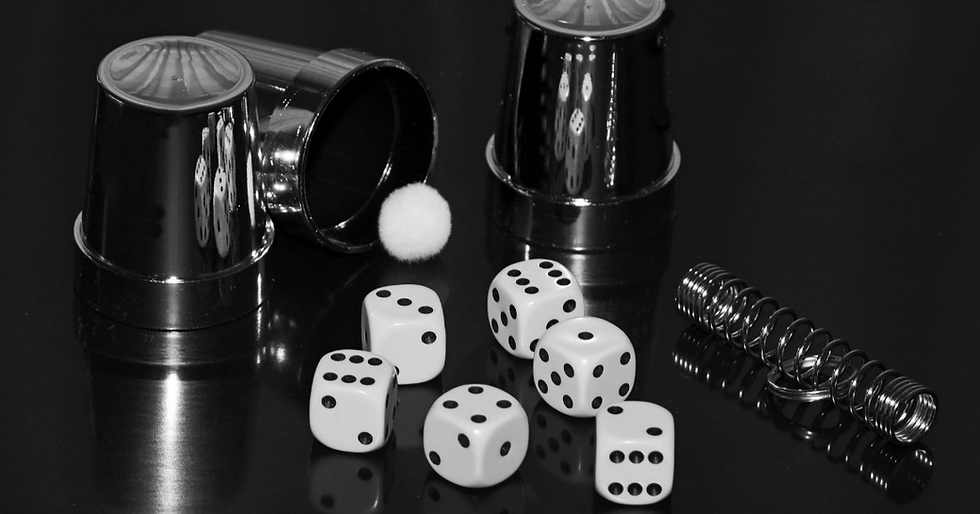
Sadly, evidence (like most other systems) can be gamed and made misleading. Entire books have been written on how to lie with statistics and how to analyze studies. These are worth reading but in the interim here are a few fast tips that will save you a world of time when analyzing data.
Be wary of popular science summaries of research. Popular science has a different goal than normal science: its goal is to be read more than to be accurate. This tends to lead popular science to make much stronger claims than what a proper systematic evaluation would show. A study claiming that “Chocolate is healthy for you” is far more interesting than one that says “Some minor negative effects and some minor positive effects found in mice eating chocolate.” Whenever somethings starts to seem a bit too polished or shocking it often ends up to be stronger claims than the original science. This sort of pop science is also extremely common on charities websites.
Be wary of abstracts. An abstract is a handy tool for quickly understanding a study, but much like pop science it can often overstate the claim. For studies that are really important to your charity's impact you will have to dig a bit deeper.
Check effect sizes. "Statistically significant" is what many studies focus on, but unfortunately this number is just not enough to make important claims. An effect size shows the magnitude or importance of the effect. For example, it could be statistically true that eating a certain food reduces cancer risk. However, if the effect size is extremely small, it would likely not be worth changing your diet. With respect to charitable interventions, it might be true that clean water reduces diseases, but the really important question is: by how much does it reduce them – i.e., what is the effect size?
Do a multiple analyze correction: A huge mistake a ton of researchers make is to measure and analyze a large number of effects without statistically taking this into account. A simple example of how this could go wrong: if you are measuring the effect of jelly beans on cancer and you measure and analyze a single variable, you will find jelly beans have no effect. However, if you break the jelly beans down into groups by color and test each color individually, you will have a much larger chance of finding an effect by pure chance even if no effect exists. There are very simple ways to fix this problem, such as using a Bonferroni correction. If a study measures a lot of factors it's worth using something like this to see if the effect holds up.
Do a multiple study correction: The same problem described above can also happen with multiple studies. If you see a single study that says a claim for example that chocolate is healthy for you make sure to check the other studies to make sure that their are not 100 studies saying it's bad for you and 1 saying it's good (in those sort of cases it's very likely the single study is wrong not the 100 previous ones).
Replicability: In general be careful with how often you expect a study to replicate. Many people assume that if it's statistically significant at 95%, it will replicate 95% of the time in future studies. This is very far from true. In fact, a p-value of 0.04 means that it'll only replicate on average 25% of the time. This is part of the reason why multiple studies converging on the same answer is so important.
Regression to the mean: Effects generally get weaker as they get studied more; this is a universal statistical phenomenon. Extreme initial measurements tend to become closer to average the next time they are studied. This should make you very cautious of single pieces of evidence that show unbelievable effects.
Comments